Keynote Speakers
Fabrizio Silvestri
Facebook, UK
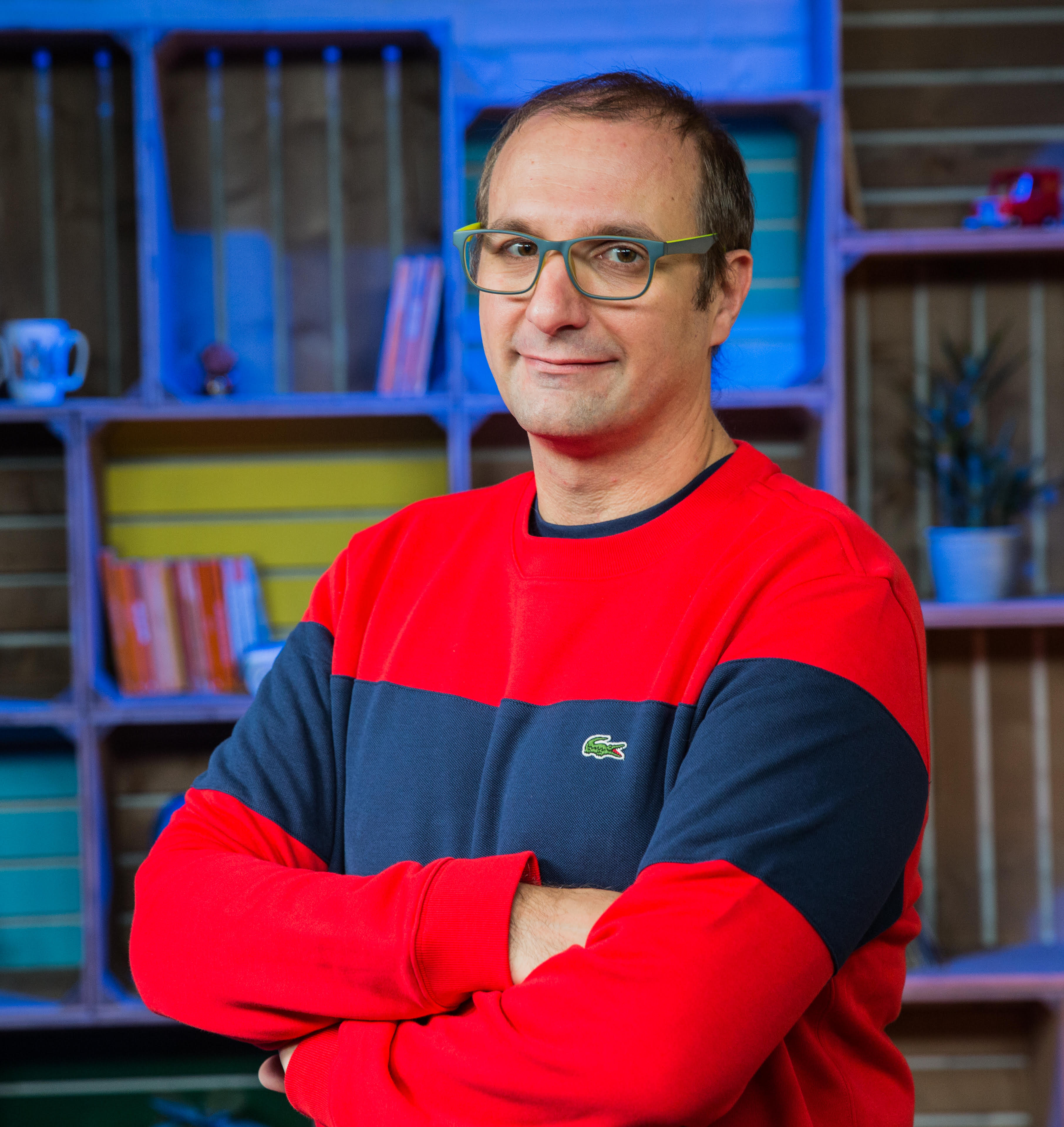
Title:Applications of Similarity Search to Socially Relevant Problems
Abstract: The Facebook AI team in London deals with applying artificial intelligence techniques to address societal problems such as the spread of online misinformation, or the integrity of election processes around the world. To do so, we have developed throughout the last years a set of tools that exploit similarity search technologies to efficiently and effectively run a very high number of classification tasks on a massive set of data.
In this talk, we are going to review some of the problems we have studied in the last year and we are going to show some of the solutions we have adopted in order to make the system run efficiently. We are also going to showcase some details of an internal project that uses similarity search as a core operation to allow efficient and effective inference operations.
Bio: Fabrizio Silvestri is a Software Engineer at Facebook London in the Search Systems team. His interests are in web search in general and in particular his specialization is building systems to better interpret queries from search users. Prior to Facebook, Fabrizio was a principal scientist at Yahoo where he has worked on sponsored search and native ads within the Gemini project. Fabrizio holds a Ph.D. in Computer Science from the University of Pisa, Italy where he studied problems related to Web Information Retrieval with particular focus on Efficiency related problems like Caching, Collection Partitioning, and Distributed IR in general.
Cong Yu
Google Research
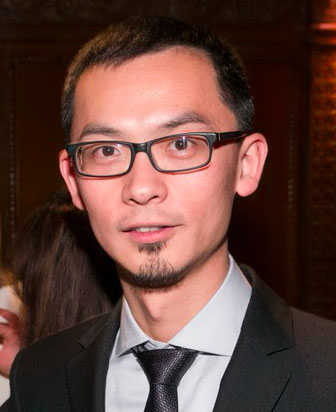
Google Research
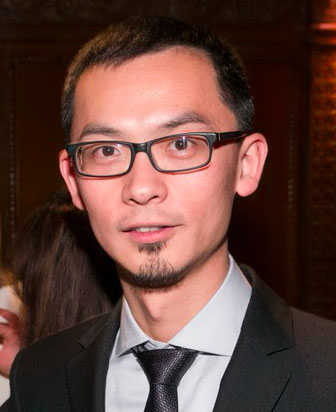
Title:Document Association for Online Information Quality
Abstract: Improving information quality online is a challenging task that requires collaboration among journalists, researchers, platforms, and standards communities. The ClaimReview structured data (http://schema.org/ClaimReview) is a successful example of such collaborations and powers various fact check features for Google. In the first part of this talk, I will briefly describe the genesis of ClaimReview and its role in combating online misinformation. This sets up the context in which advanced similarity techniques will play an increasingly important role for online information quality. In particular, I will describe our recent technical work on leveraging AI techniques to identify associated documents for claims made within the fact-checking articles. Significant challenges still remain, and, through this talk, I hope to inspire the SISAP community to take on those challenges.
Bio:
Cong Yu is a research scientist and manager at Google Research in New York City and leads the Structured Data Research Group. The group’s mission is to understand and leverage structured data on the Web to enhance user experience for Google products and has been responsible for several impactful products such as WebTables, Structured Snippets, and Fact Checking at Google. Currently, his group focuses on technical research for news and has been partnering with journalists and policy advisors to combat online misinformation and improve news consumption.
His research interests are structured data exploration and mining, computational journalism, social content analysis and recommendation, human-scalable information management, and applied ML and NLP. He was a conference keynote speaker for VLDB 2019 and twice served as an industrial program co-chair for VLDB (2013 and 2018). Outside of Google, he occasionally teaches at NYU Courant's Department of Computer Science. Before Google, Cong was a Research Scientist at Yahoo! Research, also in NYC. He has a PhD from the University of Michigan, Ann Arbor, advised by Prof. H.V. Jagadish.
Alexander Tuzhilin
New York University, USA
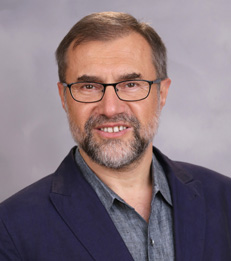
Title: On Similarity Measures in Recommender Systems
Abstract: Measures of similarity between users and between items to be recommended to the users lie at the core of many recommendation algorithms, and numerous metrics have been proposed in the recommender systems field since its inception. This talk will explore evolution of various similarity-based measures from the initial class of rating-based measures to the more recently proposed latent metrics and the metric learning methods. We will also explore possible future research directions and novel applications of similarity measures in recommender systems.
Bio: Alexander Tuzhilin is Leonard N. Stern Professor of Business in the Department of Technology, Operations and Statistics at the Stern School of Business, NYU. His research interests include personalization, recommender systems, machine learning and AI. He has produced over 140 research publications on these and related topics. Professor Tuzhilin has served on the organizing committees of numerous conferences, including as the Program and the General Chair of the IEEE International Conference on Data Mining (ICDM), and as the Program and the Conference Chair of the ACM Conference on Recommender Systems (RecSys). He served on the editorial boards of several journals, including as the Editor-in-Chief of the ACM Transactions on Management Information Systems. Professor Tuzhilin has received Ph.D. in Computer Science from the Courant Institute of Mathematical Sciences, NYU and Masters in Engineering Economics from Stanford University.